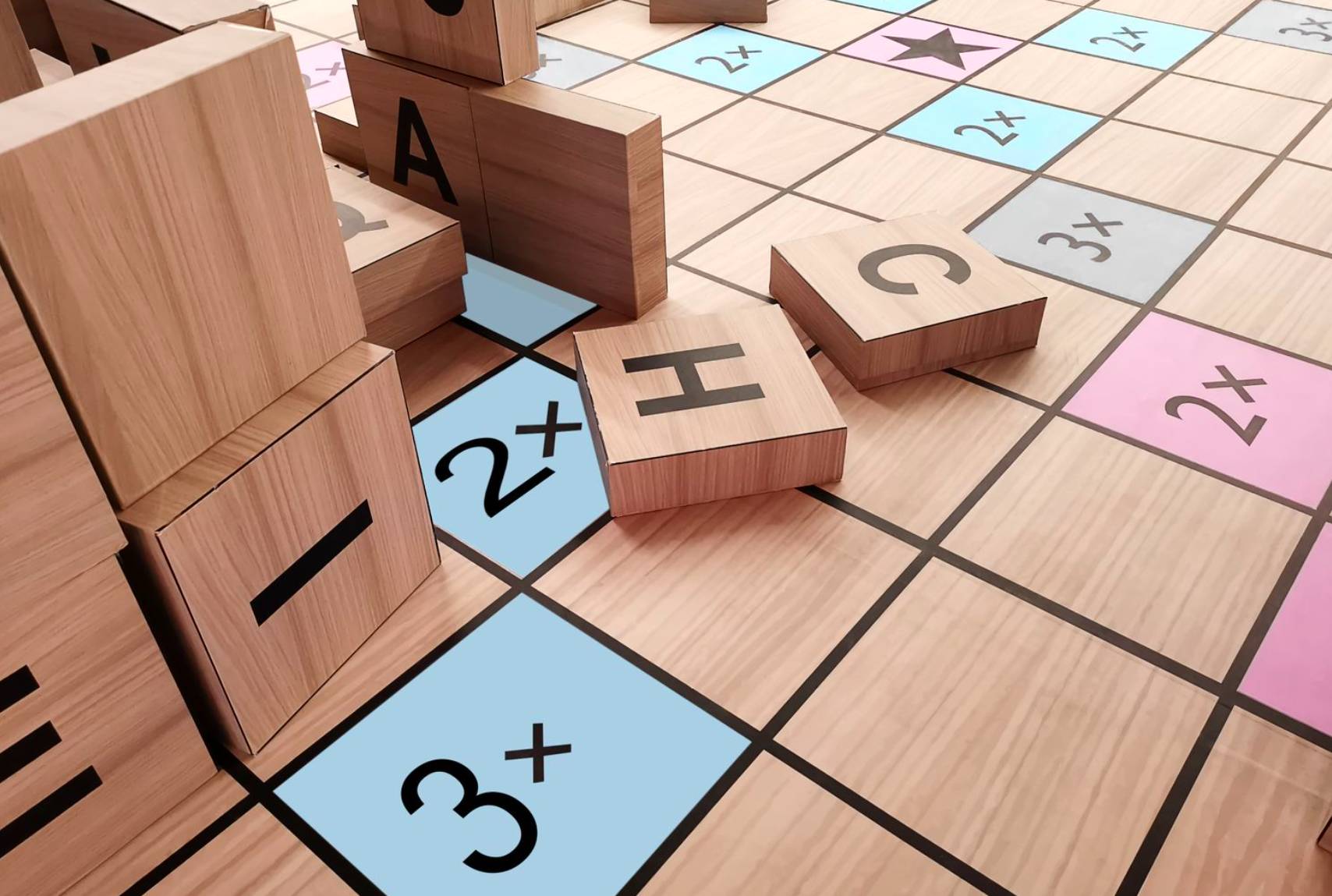
The Importance of Explainable AI
In today’s rapidly evolving technological landscape, Artificial Intelligence (AI) has become a cornerstone of innovation, driving advancements in various sectors. However, as AI systems grow more complex, the need for transparency and understanding becomes increasingly critical. This is where Explainable AI (XAI) comes into play. In this blog post, we will delve into the importance of explainable AI, its benefits, challenges, and actionable strategies for implementation.
What is Explainable AI?
Explainable AI refers to AI systems that provide human-understandable justifications for their decisions and actions. Unlike traditional “black box” AI models, which offer little insight into their inner workings, XAI aims to make the decision-making process more transparent and comprehensible. This transparency is crucial for building trust and ensuring ethical AI deployment.
Why is Explainable AI Important?
The importance of explainable AI cannot be overstated. Here are several key reasons why XAI is essential:
1. Building Trust
Trust is fundamental in any human-machine interaction. According to a MIT Sloan Management Review survey, 82% of executives believe that AI must be explainable to gain user trust. When users understand how AI arrives at its conclusions, they are more likely to trust and adopt the technology.
2. Ensuring Accountability
Accountability is another critical aspect. In sectors like healthcare, finance, and law enforcement, AI decisions can have profound impacts on individuals’ lives. Explainable AI allows stakeholders to trace the reasoning behind decisions, making it easier to identify and rectify errors, biases, or unethical practices.
3. Enhancing Regulatory Compliance
Regulatory bodies are increasingly emphasizing the need for transparency in AI systems. For instance, the European Union’s General Data Protection Regulation (GDPR) mandates that individuals have the right to an explanation for automated decisions that affect them. Explainable AI helps organizations comply with such regulations, avoiding legal repercussions and maintaining their reputation.
Challenges in Implementing Explainable AI
While the benefits of explainable AI are clear, implementing it comes with its own set of challenges:
1. Complexity of AI Models
Modern AI models, especially deep learning algorithms, are incredibly complex. Simplifying these models to make them explainable without losing their accuracy and efficiency is a significant challenge. Researchers and developers must strike a balance between transparency and performance.
2. Trade-offs Between Accuracy and Explainability
In some cases, enhancing the explainability of an AI model may lead to a reduction in its accuracy. Organizations must carefully assess the trade-offs and determine the optimal level of explainability that aligns with their goals and requirements.
3. Diverse Stakeholder Needs
Different stakeholders, such as data scientists, end-users, and regulatory authorities, have varying needs and expectations regarding AI explanations. Designing explanations that cater to these diverse needs adds another layer of complexity to the implementation process.
Actionable Tips for Implementing Explainable AI
Despite the challenges, there are several strategies that organizations can adopt to implement explainable AI effectively:
1. Adopt Interpretable Models
Whenever possible, opt for interpretable models such as decision trees, linear regression, or rule-based systems. These models inherently offer transparency and are easier to explain compared to complex neural networks.
2. Use Post-Hoc Explanation Techniques
Post-hoc techniques provide explanations for complex models after they have made decisions. Techniques like LIME (Local Interpretable Model-agnostic Explanations) and SHAP (SHapley Additive exPlanations) can help in understanding the model’s behavior without compromising its performance.
3. Foster a Culture of Transparency
Encourage a culture of transparency within your organization. This involves fostering open communication, providing training on explainable AI, and promoting ethical AI practices. A transparent culture ensures that explainability is a priority at every stage of AI development.
4. Engage with Stakeholders
Engage with all relevant stakeholders to understand their needs and expectations. Conduct workshops, surveys, and feedback sessions to gather insights and tailor explanations accordingly. This collaborative approach ensures that the explanations are meaningful and useful to all parties involved.
5. Leverage AI Governance Frameworks
Implement AI governance frameworks that outline best practices for explainable AI. These frameworks should include guidelines for model selection, explanation techniques, and monitoring mechanisms to ensure ongoing transparency and accountability.
Real-world Examples of Explainable AI
Several organizations and industries are already leveraging explainable AI to enhance their operations:
1. Healthcare
In healthcare, explainable AI is used to improve diagnostic accuracy and treatment plans. For example, IBM Watson Health provides clinicians with understandable insights into patient data, helping them make informed decisions. By explaining how it arrives at its recommendations, Watson Health builds trust and facilitates better patient outcomes.
2. Finance
The finance sector relies on explainable AI to detect fraud and assess credit risk. Zest AI, a leading fintech company, uses explainable machine learning models to evaluate loan applications. By providing clear explanations for approval or denial decisions, Zest AI ensures regulatory compliance and fosters customer trust.
3. Law Enforcement
Law enforcement agencies are adopting explainable AI to enhance transparency and accountability in their operations. For instance, PredPol, a predictive policing tool, uses explainable algorithms to forecast crime hotspots. By explaining the factors influencing its predictions, PredPol enables officers to make data-driven decisions while maintaining public trust.
The Future of Explainable AI
The future of explainable AI looks promising, with ongoing research and advancements aimed at making AI more transparent and understandable. As AI continues to evolve, the demand for explainability will only grow stronger. Organizations that prioritize explainable AI will be better positioned to harness the full potential of AI while ensuring ethical and responsible use.
Conclusion
Explainable AI is not just a buzzword; it is a fundamental requirement for building trust, ensuring accountability, and complying with regulations in today’s AI-driven world. By adopting interpretable models, leveraging post-hoc techniques, fostering a culture of transparency, engaging with stakeholders, and implementing AI governance frameworks, organizations can effectively navigate the challenges and reap the benefits of explainable AI. As we move forward, explainable AI will play a pivotal role in shaping the future of AI, making it more transparent, ethical, and trustworthy.