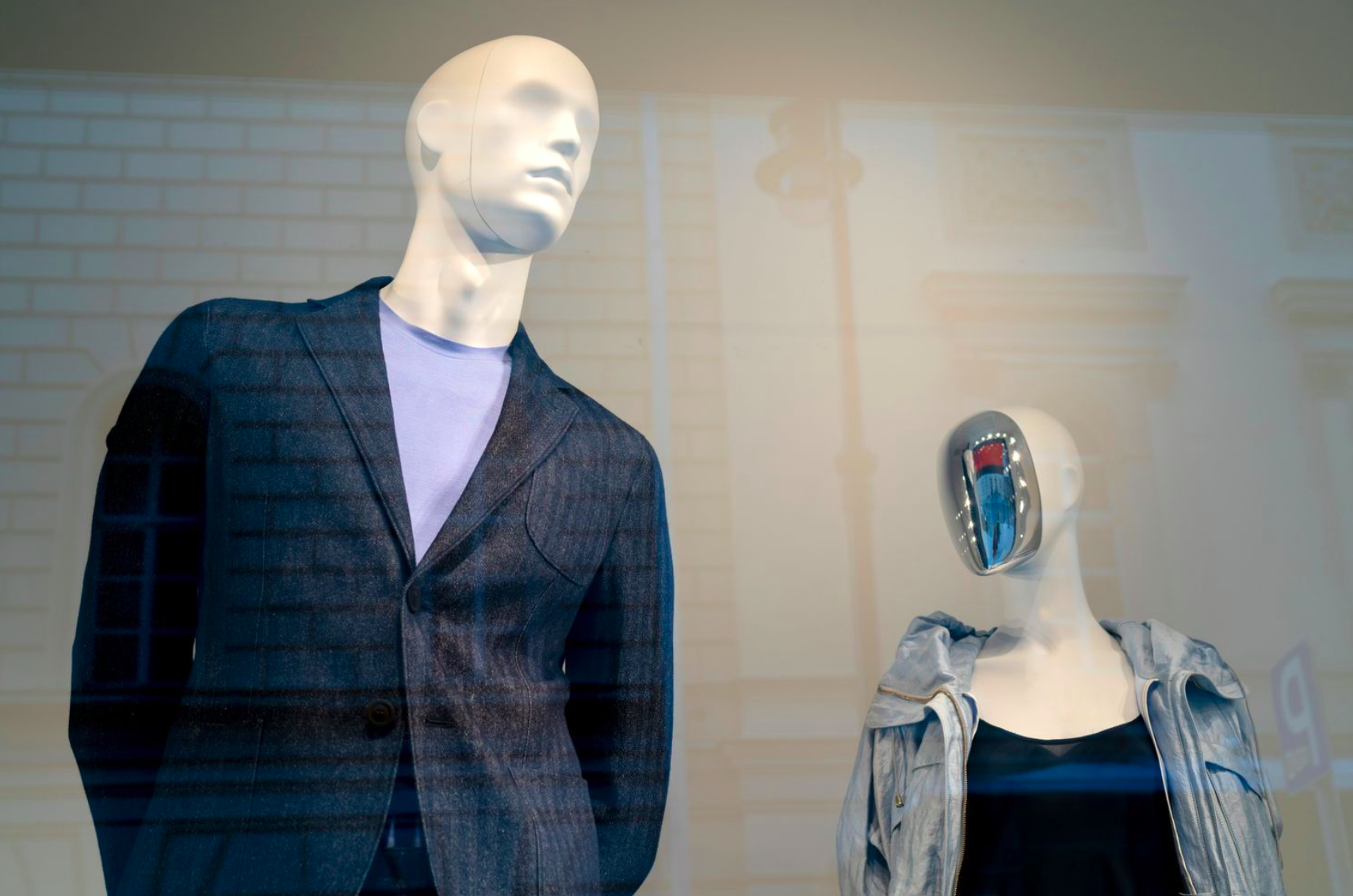
How to Address Bias in AI Systems
Artificial Intelligence (AI) has become an integral part of our lives, from powering search engines to making personalized recommendations. However, AI systems are not immune to biases, which can lead to unfair outcomes and perpetuate existing inequalities. Addressing bias in AI systems is crucial to building fair, ethical, and trustworthy technologies. In this blog post, we will explore how to identify, understand, and mitigate bias in AI systems.
Understanding Bias in AI Systems
Bias in AI systems can manifest in various forms, including gender, racial, and socioeconomic biases. These biases often stem from the data used to train AI models, the algorithms themselves, and the people involved in developing these systems.
Types of Bias
There are several types of bias that can affect AI systems:
- Data Bias: This occurs when the training data is unrepresentative or contains historical biases.
- Algorithmic Bias: This happens when the algorithms used in AI systems inherently favor certain groups over others.
- Human Bias: This is introduced when human developers unintentionally incorporate their own biases into the AI system.
Impact of Bias in AI Systems
Bias in AI systems can have significant consequences, including:
- Discrimination: Biased AI systems can lead to discriminatory practices in areas such as hiring, lending, and law enforcement.
- Loss of Trust: Users may lose trust in AI systems if they perceive them as unfair or biased.
- Reinforcement of Inequality: Biases in AI can perpetuate and even exacerbate existing social inequalities.
Identifying Bias in AI Systems
To address bias in AI systems, it is essential first to identify its presence. Here are some strategies to help detect bias:
Data Audits
Conducting regular data audits can help identify biases in the training data. This involves examining the data for any unrepresentative samples or historical biases. For example, if an AI system is being trained to recognize faces, it is crucial to ensure that the training data includes a diverse set of faces from different racial and ethnic backgrounds.
Algorithmic Audits
Algorithmic audits involve evaluating the algorithms used in AI systems to detect any inherent biases. This can be done by analyzing the decision-making processes of the algorithms and examining their outputs for any patterns of bias. For instance, if an AI system is used for hiring, it is essential to ensure that the algorithms do not favor certain genders or ethnicities over others.
User Feedback
Collecting user feedback can provide valuable insights into potential biases in AI systems. Users can report any unfair or biased outcomes they experience, which can then be investigated and addressed. For example, if users report that a recommendation system consistently suggests products that are not relevant to their preferences, it may indicate a bias in the system.
Mitigating Bias in AI Systems
Once bias has been identified, the next step is to mitigate it. Here are some actionable tips to help reduce bias in AI systems:
Diversify Training Data
One of the most effective ways to mitigate bias is to diversify the training data. Ensuring that the data used to train AI models is representative of the entire population can help reduce biases. For example, if an AI system is used for medical diagnosis, it is crucial to include data from patients of different ages, genders, and ethnicities to ensure that the system performs well for all groups.
Regularly Update Models
AI models should be regularly updated with new data to ensure that they remain accurate and unbiased. This involves continuously monitoring the performance of the models and retraining them with updated data to correct any biases that may have emerged. For instance, if an AI system is used for predicting loan defaults, it is essential to update the model with recent financial data to ensure that it remains fair and accurate.
Implement Fairness Constraints
Fairness constraints can be incorporated into the algorithms to ensure that they produce fair outcomes. This involves setting specific criteria that the algorithms must meet to ensure that they do not favor certain groups over others. For example, if an AI system is used for hiring, fairness constraints can be implemented to ensure that the system does not discriminate against candidates based on their gender or ethnicity.
Increase Transparency
Transparency is crucial for building trust and accountability in AI systems. Providing clear explanations of how AI systems make decisions and ensuring that users can understand and challenge these decisions can help reduce bias. For example, if an AI system is used for credit scoring, providing users with detailed information about how their credit scores are calculated can help them understand and address any potential biases.
Promote Diversity in AI Development Teams
Having diverse teams involved in the development of AI systems can help reduce biases. Diverse teams bring different perspectives and experiences, which can help identify and address potential biases that may be overlooked by homogenous teams. For example, if an AI system is being developed for healthcare, having a team that includes individuals from different medical specialties, genders, and ethnicities can help ensure that the system is fair and unbiased.
Examples of Bias in AI Systems
Here are some real-world examples of bias in AI systems:
Facial Recognition
Facial recognition systems have been found to exhibit racial and gender biases. Studies have shown that these systems often perform poorly on individuals with darker skin tones and women compared to individuals with lighter skin tones and men. For instance, a study conducted by MIT Media Lab found that facial recognition systems had error rates of up to 34.7% for dark-skinned women, compared to 0.8% for light-skinned men.
Hiring Algorithms
AI systems used for hiring have also been found to exhibit biases. For example, Amazon’s hiring algorithm was found to discriminate against female candidates by favoring resumes that included male-dominated terms and experiences. As a result, the company had to scrap the algorithm and develop a new system to address the bias.
Predictive Policing
Predictive policing systems, which use AI to predict criminal activity, have been criticized for perpetuating racial biases. These systems often rely on historical crime data, which can reflect existing biases in law enforcement practices. For example, a study by the AI Now Institute found that predictive policing systems disproportionately targeted minority communities, leading to over-policing and increased scrutiny of these communities.
Conclusion
Addressing bias in AI systems is essential for building fair, ethical, and trustworthy technologies. By understanding the different types of bias, identifying their presence, and taking actionable steps to mitigate them, we can create AI systems that serve all individuals equitably. Diversifying training data, regularly updating models, implementing fairness constraints, increasing transparency, and promoting diversity in development teams are all crucial strategies for reducing bias in AI systems. As we continue to advance in the field of AI, it is our responsibility to ensure that these systems are fair and just for everyone.