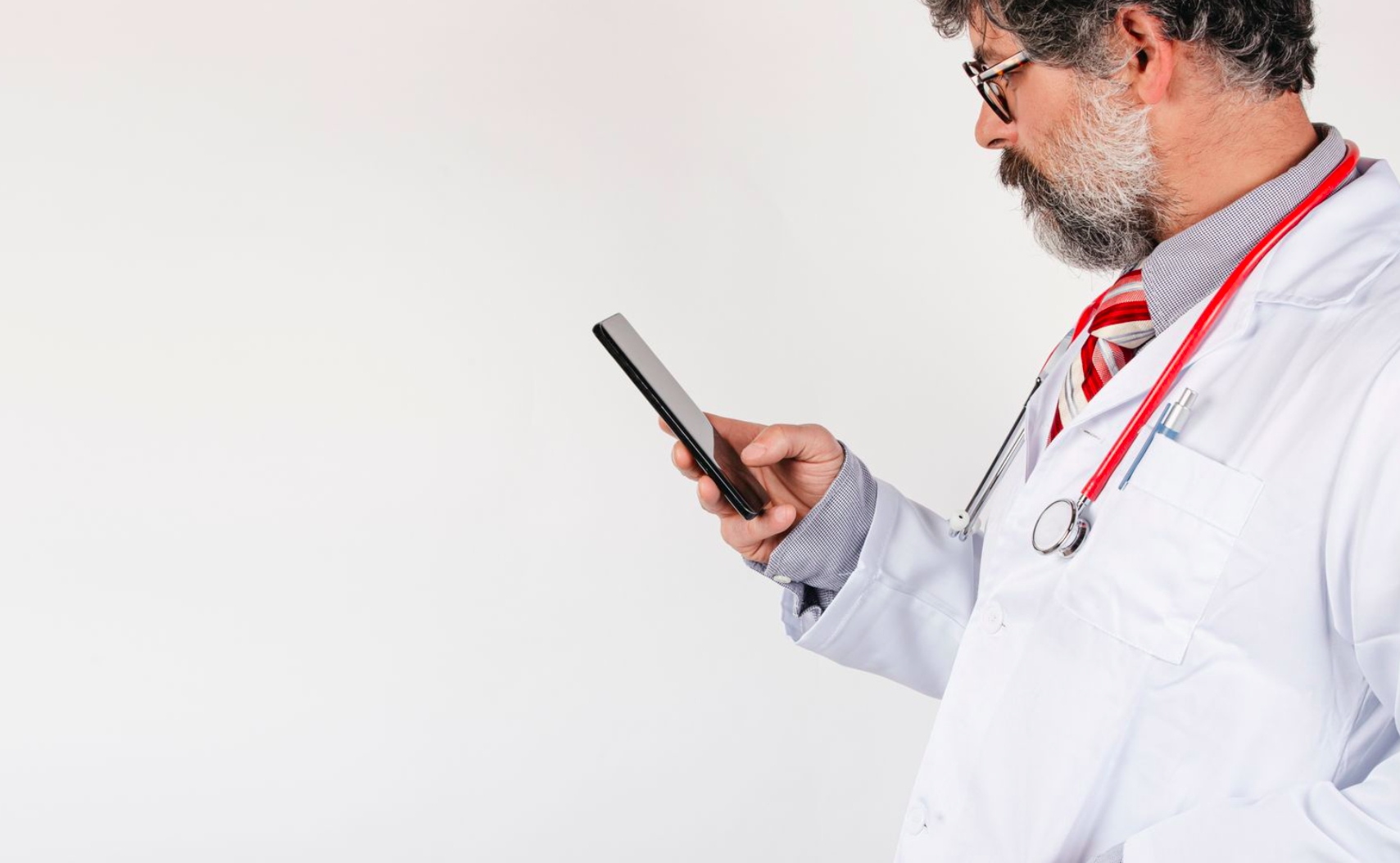
AI in Healthcare: Predictive Analytics and Patient Care
The integration of Artificial Intelligence (AI) in healthcare is no longer a futuristic concept but a present-day reality. Among the myriad applications of AI, predictive analytics stands out as a game-changer in patient care. This article delves into how AI-driven predictive analytics is transforming the healthcare landscape, enhancing patient outcomes, and streamlining operations.
What is Predictive Analytics in Healthcare?
Predictive analytics involves the use of historical data, machine learning algorithms, and statistical techniques to predict future outcomes. In healthcare, predictive analytics can forecast disease outbreaks, patient admissions, and treatment responses, among other things. This powerful tool enables healthcare providers to make data-driven decisions, ultimately improving patient care.
The Role of AI in Predictive Analytics
AI enhances predictive analytics by processing large datasets quickly and accurately. Machine learning algorithms can identify patterns and correlations that may be imperceptible to human analysts. By leveraging AI, healthcare organizations can gain deeper insights into patient data, enabling more accurate predictions and personalized care plans.
Benefits of AI-Driven Predictive Analytics
Implementing AI in predictive analytics offers numerous benefits:
- Early Disease Detection: AI can analyze patient data to identify early signs of diseases like cancer, diabetes, and heart conditions, allowing for timely intervention.
- Personalized Treatment Plans: AI enables the customization of treatment plans based on individual patient data, improving the effectiveness of interventions.
- Resource Optimization: Predictive analytics can forecast patient admissions and resource needs, helping hospitals manage staff and supplies more efficiently.
- Reduced Readmission Rates: By identifying patients at risk of readmission, healthcare providers can implement preventive measures, reducing readmission rates and associated costs.
Real-World Examples of AI in Predictive Analytics and Patient Care
Several healthcare organizations have successfully implemented AI-driven predictive analytics to enhance patient care:
Example 1: Cleveland Clinic
The Cleveland Clinic uses predictive analytics to identify patients at risk of heart failure. By analyzing electronic health records (EHRs) and other data, the clinic can predict which patients are likely to develop heart failure and intervene early. As a result, the clinic has seen a significant reduction in heart failure admissions.
Example 2: Kaiser Permanente
Kaiser Permanente employs AI to predict patient readmissions. The health organization uses machine learning algorithms to analyze patient data and identify those at high risk of readmission. This information allows healthcare providers to implement targeted interventions, reducing readmission rates and improving patient outcomes.
Example 3: Mount Sinai Health System
Mount Sinai Health System has developed an AI-powered predictive analytics platform called “Deep Patient.” This platform analyzes EHRs to predict various diseases and conditions. Deep Patient has demonstrated remarkable accuracy in predicting diseases such as liver cancer and psychiatric disorders, enabling early intervention and better patient care.
Challenges and Limitations of AI in Predictive Analytics
While AI-driven predictive analytics offers significant benefits, it is not without challenges:
Data Privacy and Security
Handling sensitive patient data requires stringent privacy and security measures. Healthcare organizations must comply with regulations such as HIPAA (Health Insurance Portability and Accountability Act) to protect patient information. Implementing robust cybersecurity measures is essential to prevent data breaches and maintain patient trust.
Data Quality and Integration
For predictive analytics to be effective, the quality and completeness of data are crucial. Incomplete or inaccurate data can lead to incorrect predictions and suboptimal patient care. Additionally, integrating data from various sources, such as EHRs, wearable devices, and lab results, can be challenging but is necessary for comprehensive analysis.
Algorithm Bias
AI algorithms can be biased if trained on unrepresentative or biased data. This can lead to disparities in healthcare outcomes. It is essential to ensure that training data is diverse and representative of the patient population to minimize bias and promote equitable care.
Future Prospects of AI in Predictive Analytics and Patient Care
The future of AI in predictive analytics and patient care looks promising. Advancements in AI technologies, such as deep learning and natural language processing, will further enhance predictive capabilities. Here are some anticipated future developments:
Integration with Wearable Devices
Wearable devices, such as smartwatches and fitness trackers, generate vast amounts of health data. Integrating this data with AI-driven predictive analytics can provide real-time insights into a patient’s health, enabling proactive care and early intervention.
Telemedicine and Remote Monitoring
The COVID-19 pandemic has accelerated the adoption of telemedicine and remote monitoring. AI-powered predictive analytics can enhance remote patient monitoring by analyzing real-time data and alerting healthcare providers to potential issues, ensuring timely intervention and continuous care.
Personalized Medicine
The future of healthcare lies in personalized medicine, where treatment plans are tailored to individual patients based on their unique genetic makeup, lifestyle, and medical history. AI-driven predictive analytics will play a crucial role in developing personalized treatment plans, improving patient outcomes, and reducing healthcare costs.
Actionable Tips for Implementing AI in Predictive Analytics
Healthcare organizations looking to implement AI-driven predictive analytics can follow these actionable tips:
Invest in Quality Data
Ensure that your data is accurate, complete, and up-to-date. Invest in data management systems and processes to maintain high-quality data, which is essential for effective predictive analytics.
Collaborate with AI Experts
Partner with AI experts and data scientists to develop and implement predictive analytics models. Their expertise will be invaluable in creating accurate and reliable predictions.
Ensure Data Privacy and Security
Implement robust data privacy and security measures to protect patient information. Compliance with regulations such as HIPAA is crucial to maintaining patient trust and avoiding legal issues.
Continuously Monitor and Improve
Regularly monitor the performance of your predictive analytics models and make necessary adjustments. Continuous improvement ensures that your models remain accurate and effective over time.
Educate and Train Staff
Provide training to healthcare staff on the use of AI-driven predictive analytics tools. Ensuring that staff are comfortable with these tools will enhance their effectiveness and improve patient care.
Conclusion
AI-driven predictive analytics holds immense potential to revolutionize patient care in the healthcare industry. By enabling early disease detection, personalized treatment plans, and resource optimization, AI can significantly enhance patient outcomes and operational efficiency. While challenges such as data privacy, quality, and algorithm bias exist, the future prospects of AI in healthcare are promising. By investing in quality data, collaborating with AI experts, and ensuring continuous improvement, healthcare organizations can harness the power of AI to provide better patient care and transform the healthcare landscape.