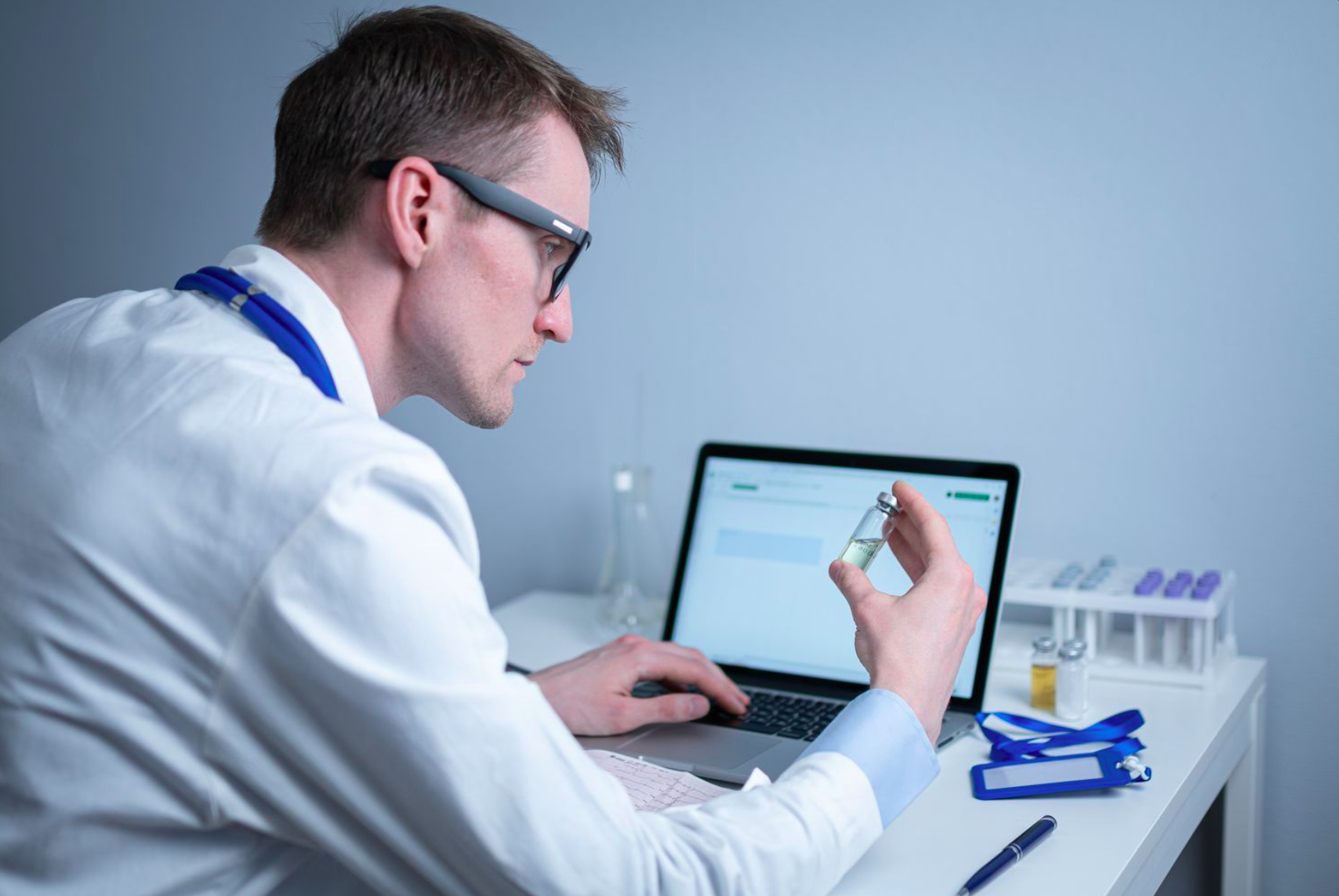
The Challenges of AI in Medical Diagnosis
Artificial Intelligence (AI) has revolutionized various sectors, and healthcare is no exception. The integration of AI in medical diagnosis promises to enhance accuracy, efficiency, and patient outcomes. However, this transformation is not without its challenges. In this blog post, we will delve into the complexities and hurdles that come with implementing AI in medical diagnosis.
Introduction to AI in Medical Diagnosis
AI in medical diagnosis involves the use of complex algorithms and machine learning models to analyze medical data and assist healthcare professionals in identifying diseases. From early detection of cancer to predicting patient outcomes, AI has a wide range of applications in the medical field. According to a report by Accenture, AI applications could create $150 billion in annual savings for the U.S. healthcare economy by 2026.
The Accuracy and Reliability Challenge
One of the primary challenges in using AI for medical diagnosis is ensuring accuracy and reliability. While AI algorithms can process vast amounts of data quickly, they are only as good as the data they are trained on. Inaccurate or biased data can lead to incorrect diagnoses, which can have serious implications for patient care.
Data Quality and Bias
AI models rely heavily on training data to make predictions. If the training data is flawed or biased, the model’s predictions will also be flawed. For instance, a study published in the journal Science found that an AI system used to predict health outcomes was biased against black patients. This highlights the importance of using high-quality, diverse data sets to train AI models.
Example: IBM Watson’s Setback
IBM’s Watson for Oncology was once hailed as a breakthrough in AI-driven cancer diagnosis. However, it faced setbacks due to inaccurate recommendations and a lack of reliable data. This case underscores the need for rigorous validation and continuous monitoring of AI systems in healthcare.
Ethical and Legal Challenges
The integration of AI in medical diagnosis also raises ethical and legal concerns. These challenges must be addressed to ensure that AI is used responsibly and ethically in healthcare.
Patient Privacy
AI systems require access to vast amounts of patient data to function effectively. This raises concerns about patient privacy and data security. Healthcare providers must ensure that patient data is anonymized and protected to prevent unauthorized access and breaches.
Liability and Accountability
Determining liability in cases where an AI system makes an incorrect diagnosis is another significant challenge. Who is responsible if an AI-driven diagnosis leads to patient harm? Is it the healthcare provider, the AI developer, or both? Clear regulations and guidelines are needed to address these questions.
Actionable Tip: Healthcare providers should work closely with legal experts to establish clear protocols and guidelines for the use of AI in medical diagnosis.
Integration with Existing Systems
Integrating AI systems with existing healthcare infrastructure can be a complex process. Many healthcare facilities still rely on outdated systems that are not compatible with advanced AI technologies.
Interoperability
Interoperability is crucial for the seamless integration of AI systems in healthcare. AI solutions must be compatible with Electronic Health Records (EHR) and other medical systems to ensure smooth data exchange and functionality.
Training and Adoption
Healthcare professionals need to be adequately trained to use AI systems effectively. Resistance to change and a lack of understanding of AI technology can hinder its adoption. Continuous education and training programs are essential to ensure that healthcare providers are comfortable and proficient in using AI tools.
Actionable Tip: Invest in comprehensive training programs for healthcare staff to ensure smooth integration and adoption of AI technologies.
Cost and Accessibility
The cost of implementing AI systems can be a barrier for many healthcare facilities, particularly in low-resource settings. Ensuring that AI technology is accessible and affordable is crucial for its widespread adoption.
Initial Investment
The initial cost of developing and deploying AI systems can be high. This includes the cost of acquiring technology, training staff, and maintaining the systems. Healthcare facilities must carefully consider the return on investment and long-term benefits of AI implementation.
Equitable Access
Ensuring equitable access to AI-driven medical diagnosis is essential to avoid widening the healthcare gap. Efforts must be made to make AI technology accessible to underserved populations and low-resource settings.
Actionable Tip: Explore partnerships and funding opportunities to reduce the financial burden of AI implementation and ensure equitable access to the technology.
Regulatory and Compliance Challenges
AI in medical diagnosis must comply with various regulatory standards and guidelines to ensure patient safety and efficacy. Navigating the regulatory landscape can be challenging for healthcare providers and AI developers.
Regulatory Approval
Obtaining regulatory approval for AI systems can be a lengthy and complex process. Regulatory bodies like the FDA in the U.S. have stringent requirements for the approval of medical devices and technologies. Ensuring compliance with these regulations is crucial for the successful deployment of AI systems.
Continuous Monitoring and Auditing
AI systems in healthcare require continuous monitoring and auditing to ensure they perform as intended. Regular audits and updates are necessary to maintain compliance with regulatory standards and to address any issues that may arise.
Actionable Tip: Establish a dedicated compliance team to navigate regulatory requirements and ensure continuous monitoring of AI systems.
Conclusion
AI has the potential to transform medical diagnosis and improve patient outcomes significantly. However, the challenges associated with its implementation cannot be ignored. By addressing issues related to accuracy, ethics, integration, cost, and regulatory compliance, healthcare providers can harness the full potential of AI while ensuring patient safety and efficacy.
As AI technology continues to evolve, it is crucial for stakeholders to stay informed and proactive in addressing these challenges. Collaboration between healthcare providers, AI developers, regulatory bodies, and policymakers will be key to unlocking the benefits of AI in medical diagnosis and creating a more efficient and effective healthcare system.