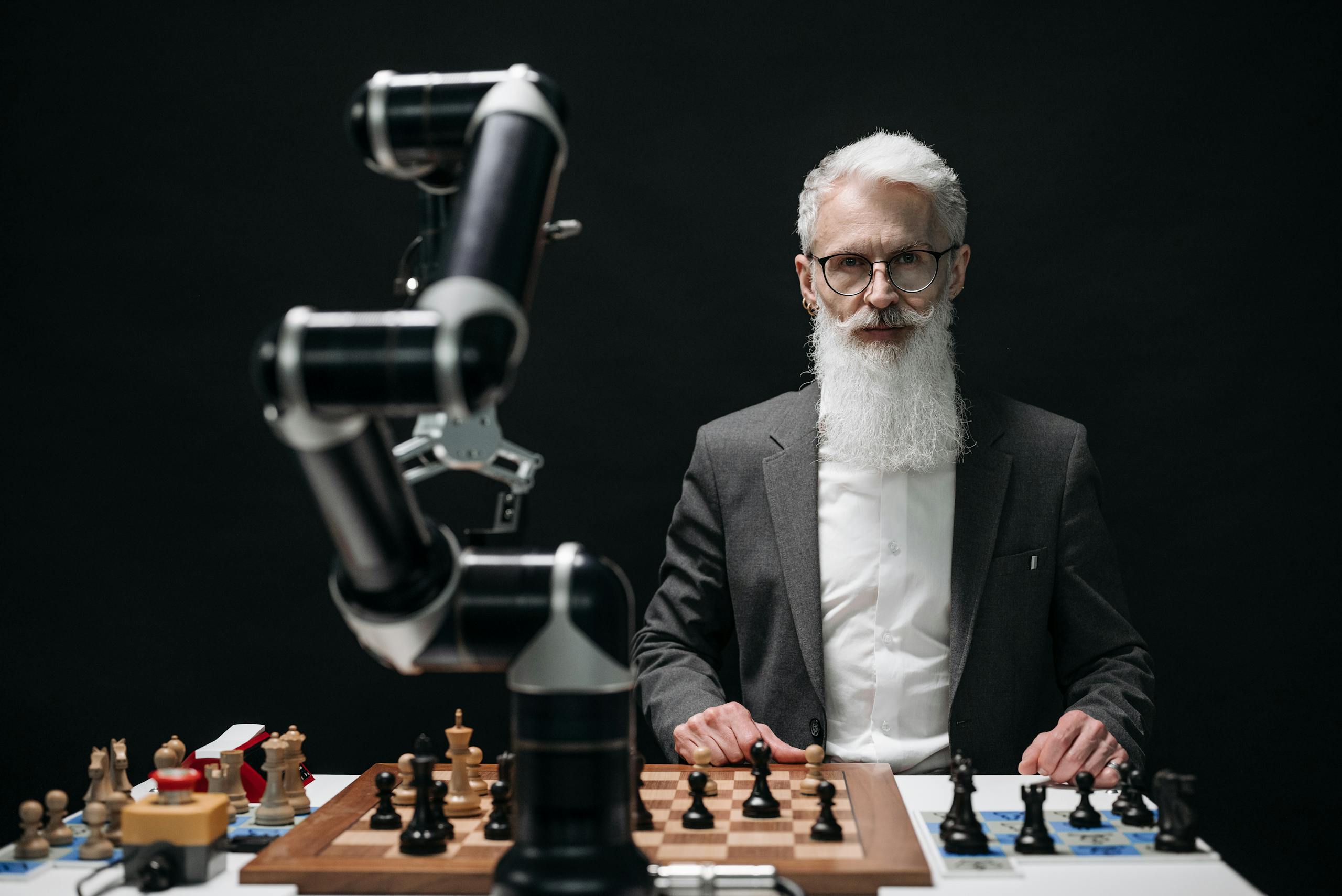
The Importance of Transparency in AI Systems
Artificial Intelligence (AI) is revolutionizing various industries, from healthcare to finance, by offering unprecedented capabilities to enhance decision-making processes. However, as AI systems become more prevalent, the need for transparency in these systems has emerged as a critical concern. Transparency in AI not only fosters trust but also ensures ethical and fair outcomes. In this blog post, we will delve into the importance of transparency in AI systems, explore its benefits, discuss the challenges, and provide actionable tips for implementing transparent AI models.
What is AI Transparency?
AI transparency refers to the clarity and openness with which an AI system operates. It involves making the inner workings of AI models understandable to stakeholders, including developers, users, and regulatory bodies. Transparent AI systems disclose their decision-making processes, the data they use, and the algorithms they employ, enabling stakeholders to comprehend, trust, and verify the outcomes produced by these systems.
Benefits of Transparency in AI Systems
Ensuring transparency in AI systems offers numerous benefits, including:
1. Building Trust with Users
Trust is paramount when it comes to AI adoption. According to a 2021 Edelman Trust Barometer report, only 61% of people trust AI technologies. Transparent AI systems help build trust by providing clear explanations about how decisions are made, enabling users to feel more confident in the system’s reliability and fairness.
2. Enhancing Accountability
Transparent AI systems make it easier to identify and address any biases or errors that may arise. By revealing the data sources and algorithms used, developers and regulatory bodies can hold AI systems accountable for their actions and ensure they comply with ethical standards and regulations.
3. Promoting Fairness and Ethical AI
Transparency helps mitigate biases and discrimination in AI systems. By understanding how decisions are made, stakeholders can identify and rectify any unfair practices, ensuring that AI systems operate in an ethical and unbiased manner. This is particularly important in sectors like hiring, lending, and law enforcement, where biased AI decisions can have significant consequences.
4. Facilitating Regulatory Compliance
With the rise of AI technologies, regulatory bodies are increasingly focusing on ensuring transparency and accountability. Transparent AI systems make it easier for organizations to comply with regulations, such as the European Union’s General Data Protection Regulation (GDPR) and the proposed Artificial Intelligence Act, which emphasize the need for explainable AI.
Challenges in Achieving AI Transparency
While the benefits of transparency are clear, achieving it in AI systems is not without challenges:
1. Complexity of AI Models
Modern AI models, especially deep learning algorithms, are inherently complex and often operate as “black boxes.” Explaining the intricate workings of these models to non-experts can be challenging. Simplifying these explanations without losing essential details is a delicate balance that developers must achieve.
2. Trade-offs between Performance and Transparency
In some cases, there may be a trade-off between the performance of an AI model and its transparency. Highly accurate models, such as deep neural networks, might be less interpretable than simpler models. Organizations need to find a balance between achieving high performance and maintaining transparency.
3. Protecting Intellectual Property
Disclosing too much information about AI models may risk revealing proprietary algorithms and trade secrets. Companies must navigate the fine line between being transparent and protecting their intellectual property.
4. Ensuring Data Privacy
Transparency requires disclosing information about the data used in AI models. However, this must be done without compromising individual privacy. Organizations need to implement robust data anonymization techniques to protect user data while maintaining transparency.
Actionable Tips for Implementing Transparent AI Models
Despite the challenges, there are several actionable steps organizations can take to enhance transparency in their AI systems:
1. Implement Explainable AI (XAI) Techniques
Explainable AI (XAI) techniques focus on creating AI models that are interpretable and understandable. Techniques like LIME (Local Interpretable Model-agnostic Explanations) and SHAP (SHapley Additive exPlanations) provide insights into how AI models make decisions, helping stakeholders understand the factors influencing the outcomes.
2. Use Transparent Algorithms
Opt for algorithms that are inherently transparent and interpretable. Decision trees, linear regression, and rule-based systems are examples of algorithms that are easier to understand and explain compared to complex neural networks.
3. Document Data Sources and Preprocessing Steps
Maintain comprehensive documentation of the data sources, preprocessing steps, and feature engineering techniques used in AI models. This documentation should be accessible to stakeholders, enabling them to understand how the data was collected, cleaned, and transformed before being used in the model.
4. Regularly Audit and Monitor AI Models
Conduct regular audits and monitoring of AI models to ensure they remain transparent, fair, and unbiased. Implement mechanisms to detect and address any biases or anomalies that may arise over time. Continuous monitoring helps maintain the integrity and trustworthiness of AI systems.
5. Foster a Culture of Transparency
Encourage a culture of transparency within the organization. Educate employees about the importance of transparency in AI and provide training on best practices for building and maintaining transparent AI models. A transparent culture promotes accountability and ethical AI practices.
Real-World Examples of Transparent AI
Several organizations are leading the way in implementing transparent AI systems. Here are a few notable examples:
1. IBM’s AI Fairness 360
IBM has developed the AI Fairness 360 toolkit, an open-source library that provides metrics to check for unwanted bias in datasets and machine learning models. The toolkit also includes algorithms to mitigate bias, ensuring that AI models are fair and transparent.
2. Google’s Model Cards
Google introduced Model Cards, which are standardized documentation for AI models. Model Cards provide information about a model’s intended use, performance, and potential biases. This transparency helps users understand the strengths and limitations of AI models and promotes responsible usage.
3. OpenAI’s GPT-3 API
OpenAI has implemented transparency measures for its GPT-3 API. The organization provides detailed documentation about the model’s capabilities, limitations, and ethical considerations. Additionally, OpenAI encourages feedback from users to continuously improve the transparency and fairness of its AI systems.
Conclusion
Transparency in AI systems is essential for building trust, ensuring accountability, promoting fairness, and complying with regulations. While achieving transparency poses challenges, organizations can implement various strategies to make their AI models more understandable and interpretable. By prioritizing transparency, organizations can harness the full potential of AI technologies while maintaining ethical and responsible practices.
As AI continues to evolve, transparency will remain a key factor in its successful and ethical adoption. By embracing transparency, we can create AI systems that are not only powerful but also trustworthy and fair.